Attention
This website is best viewed in portrait mode.
Publication Name: Hindustantimes.com
Date: December 12, 2024
Role of Edge AI in enhancing real-time data processing
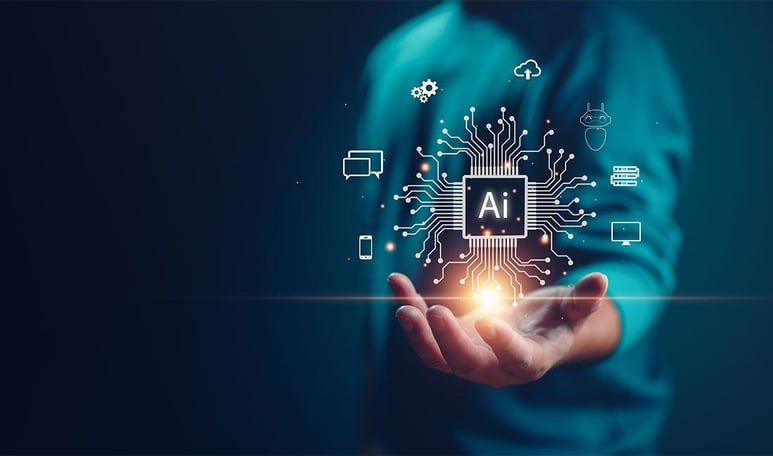
Edge Artificial Intelligence (Edge AI) has revolutionised the way data is processed in modern IoT applications, bringing real-time capabilities closer to the source of data generation—at the "edge" of networks. This shift in computing has profound implications for industries reliant on Internet of Things (IoT) devices, allowing for faster decision-making, reduced latency, and more efficient use of network resources. As we explore the role of Edge AI, it becomes clear that its ability to enhance real-time data processing is driving new possibilities for various sectors.
Traditional IoT systems have depended heavily on cloud-based models, where data is collected from edge devices, transmitted to centralised cloud servers, and then processed. While effective in some scenarios, this approach comes with inherent challenges. Latency issues arise from the distance between the edge device and the cloud server, often leading to delays that are unacceptable for time-sensitive applications. Additionally, the sheer volume of data generated by IoT devices can strain network bandwidth, causing inefficiencies and higher costs.
This is where Edge AI offers a transformative solution. By moving computation closer to where the data is generated—on the edge devices themselves—it becomes possible to perform data analysis and make decisions locally and in real-time. Instead of transmitting every piece of raw data to the cloud, edge devices can filter and process the data before sending only the most relevant information. This not only reduces network congestion but also significantly speeds up response times, which is crucial for applications like autonomous vehicles, industrial automation, and smart health care systems.
One of the major breakthroughs in Edge AI involves the development of lightweight AI models. Traditional AI models, such as deep learning networks and neural networks, are often too large and computationally heavy to run on edge devices with limited resources. These models typically require powerful GPUs or cloud-based servers to operate efficiently. However, advancements in model optimisation techniques, such as quantization and hyperparameter tuning, have enabled AI models to be compressed and optimised for edge environments. As a result, these smaller models can now run on devices with minimal processing power and memory, like microcontrollers, without compromising performance.
A key advantage of Edge AI lies in its ability to provide immediate insights through local processing. For example, in industrial settings, machines equipped with Edge AI can monitor production lines and detect anomalies in real-time. By processing the data directly on-site, these systems can identify potential faults or inefficiencies without waiting for cloud-based analysis. This allows for rapid intervention, reducing downtime and improving overall operational efficiency.
The health care industry is another sector benefiting from Edge AI. Medical devices, such as wearables and diagnostic tools, generate vast amounts of data. In scenarios where immediate action is critical—such as monitoring a patient’s vital signs—Edge AI enables real-time analysis, allowing for quicker responses to changes in a patient's condition. This capability is especially valuable in remote health care settings, where connectivity to cloud servers might be unreliable or slow.
Another significant development is the growing use of federated learning at the edge. In federated learning, multiple edge devices collaborate to train a shared AI model while keeping the data local. This decentralised approach enhances privacy and security by ensuring that sensitive data never leaves the device. Instead of sending raw data to the cloud for training, only the model updates are transmitted. This approach not only protects user privacy but also reduces the risks associated with data breaches and regulatory non-compliance.
As the edge computing ecosystem continues to mature, more sophisticated tasks can be handled locally. Emerging technologies, such as neuromorphic computing, offer even more potential by mimicking the brain’s neural architecture. These systems, designed for ultra-low-power environments, can process complex data streams with incredible speed and efficiency, making them ideal for applications that require real-time decision-making, such as robotics and autonomous systems.
However, challenges remain in fully realising the potential of Edge AI. One of the primary hurdles is the complexity of deploying AI models on resource-constrained devices. While there have been significant advancements in reducing model size and improving efficiency, many AI models still require more memory and processing power than what edge devices can provide. Additionally, maintaining model accuracy when dealing with reduced datasets at the edge can be difficult, especially in environments where the data is noisy or incomplete.
Another challenge is the variability in hardware platforms for edge computing. The diversity of edge devices—from sensors and cameras to industrial machinery—means that AI models need to be highly adaptable to different architectures. Open-source tools like Apache TVM have made strides in addressing this issue by providing frameworks that allow models to run on a wide range of hardware. This interoperability is crucial for ensuring that AI models can be deployed across various industries without extensive customisation.
Despite these challenges, the progress in Edge AI is undeniable. The ongoing evolution of processors and AI accelerators designed specifically for edge computing is making it possible to perform more complex tasks on smaller, energy-efficient devices. As Edge AI continues to develop, it is poised to unlock new applications that were previously thought to be beyond the capabilities of IoT devices.
In conclusion, the role of Edge AI in enhancing real-time data processing for modern IoT applications is pivotal. By bringing computation closer to the source of data, Edge AI reduces latency, optimises bandwidth usage, and enables faster decision-making. The convergence of AI and edge computing is driving innovation across a wide range of industries, from manufacturing and health care to agriculture and retail. While challenges remain, the advancements in lightweight AI models, federated learning, and hardware optimization are paving the way for a future where real-time, on-device intelligence becomes the norm rather than the exception.
Author: Biswajit Biswas, Chief Data Scientist, Tata Elxsi.