Attention
This website is best viewed in portrait mode.
Publication Name: Etedge-insights.com
Date: August 16, 2024
The AI Dilemma: Ethical and Regulatory Challenges in AI Adoption
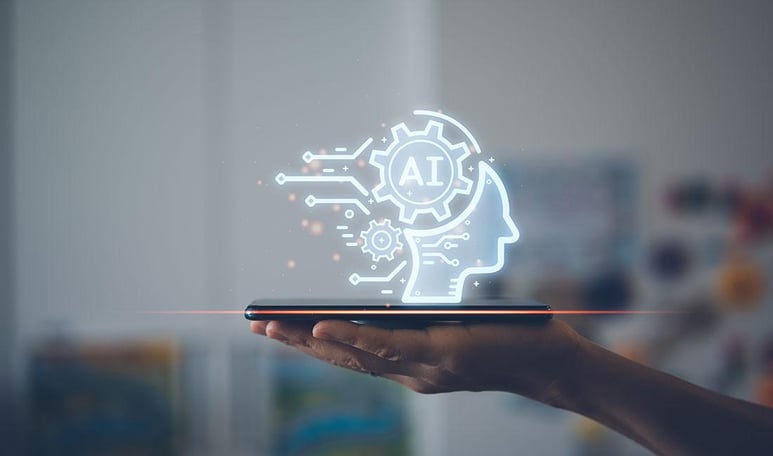
As artificial intelligence (AI) continues to revolutionize industries across the board, its integration into sectors brings both unprecedented opportunities and complex challenges. AI deployment across industries is the new norm but it brings with it several regulatory challenges that are commonly associated with data privacy, security, bias mitigation, transparency, and long-term societal impact.
Moreover, there is the fear of job displacement due to AI automation that could potentially displace human workers. There is also a heightened societal anxiety over AI’s potential to replace human creativity. With these factors in mind, the “AI dilemma” needs to be addressed, especially from an ethical and regulatory perspective.
Regulatory Challenges Associated with Data Privacy and Security
As AI systems become more sophisticated and every service is becoming hyper-personalised, they often require vast amounts of data to function effectively. Hyper-personalisation needs data to be collected and processed at individual level while at the same time without any personally identifiable information (PII) stored. This often presents a dichotomy difficult to be handled even with best use of technology. This is where regulatory laws come into the picture. Organizations are required to navigate a labyrinth of laws and regulations that vary significantly across different jurisdictions. This further includes implementing state-of-the-art encryption techniques, secure data storage protocols, and stringent access controls.
Legislation like the European Union’s General Data Protection Regulation (GDPR) provides a blueprint for comprehensive data protection laws. However, as AI capabilities grow, regulations may need to be updated to address new challenges, such as the use of synthetic data or the potential for AI to infer sensitive information from seemingly innocuous data points. At the same time, enterprises are required to be transparent about their data collection practices and provide users with clear options to control their data. There is also AI based technological support available for identifying PII from the data source and separate the same from rest of the datasets, ring fence and store them in more secure way.
Addressing Ethical Implications of AI
One of the primary ethical concerns in AI implementation is the potential for bias. AI systems, much like humans, can inherit biases from the data they’re trained on. In the context of media and news reporting, this bias can have far-reaching consequences. AI-enabled automated news gathering and reporting, including the use of digitally created news anchors, has the power to significantly influence which stories are chosen, how they are portrayed, and how they are presented to the public. If an AI system learns from historical data that certain political viewpoints or types of stories are more newsworthy, it could inadvertently skew coverage, potentially compromising the principles of fair and balanced reporting. Addressing this issue requires a multifaceted approach.
The “black box” nature of many AI systems poses another significant ethical challenge. Today, AI is increasingly influencing content curation and recommendation systems, and the lack of transparency in decision-making processes can erode user trust and raise questions about accountability. Consumers and subscribers are often left in the dark about how AI algorithms determine the content they see, from personalized news feeds to targeted advertisements. This opacity not only fuels concerns about manipulation but also makes it difficult to address issues when they arise.
Enhancing Transparency and Accountability in AI Decision-Making Process
These challenges can be overcome when organizations carefully examine the data and algorithms used in their AI systems to identify and mitigate inherent biases. This may involve diverse data sourcing, regular audits of AI outputs, and the implementation of bias detection tools. Additionally, maintaining human oversight in the overall process can help ensure that AI-generated solutions align with the established ethics and standards.
Industries leveraging AI must prioritize explainable AI (XAI) techniques that aim to make AI decision-making processes more transparent and interpretable. By providing clear explanations for AI-driven recommendations or decisions, organizations can foster trust and demonstrate accountability.
This goes beyond just by altering the tools chain (e.g, LIME, SHAP, ELI5) and bringing the visibility on feature importance, attribution and additional layers of observability. XAI is to be built layer-wise, even at the stage of data lake creating, ETL and other data processing methods so that complete transparency and traceability can be established.
Further, when enterprises publish transparency reports, it can help maintain public trust. These measures allow for external scrutiny and ensure that AI systems are operating as intended, without undue bias or unintended consequences.
Addressing the Long-Term Impact of AI
As AI becomes more deeply integrated, its long-term impact on society and culture is one of the additional factors that we need to consider. While personalization can enhance user experience, it also risks creating “filter bubbles” where individuals are exposed only to information that aligns with their existing views, potentially polarizing society. As we already know from research that significant majority of the social media users suffer phenomenon called ‘confirmation bias’, these personalisation services powered by AI adds to the problem. Further, as virtual assistants and AI-powered communication tools become more sophisticated, they may change the way we perceive and engage in human connection.
For one, an interdisciplinary collaboration between technologists, social scientists, ethicists, and policymakers can establish frameworks for assessing the societal impact of AI systems. This further brings us to the Human-AI collaboration rather than the Human-AI conflict. For instance, AI can augment human capabilities, handling routine tasks and data analysis, while humans provide creativity, emotional intelligence, and ethical judgment that AI currently lacks. To facilitate effective human-AI collaboration, organizations need to invest in reskilling and upskilling their workforce. This ensures that employees can work alongside AI systems effectively, leveraging the strengths of both human and artificial intelligence.
There is immediate need for creating roles like ‘Chief AI ethics Officer (CAIEO) in companies who are largely developing AI software and services to bring a critical oversight how the policies are implemented and commitment towards this larger goal.
In a gist, it’s imperative that we approach the development and deployment of AI with a strong ethical framework. This involves addressing biases, ensuring transparency and accountability, protecting user privacy and data security, fostering productive human-AI collaboration, and carefully considering the long-term societal impacts. By navigating these ethical considerations thoughtfully, we can leverage AI’s potential to enhance creativity, efficiency, and user experience while safeguarding human values, privacy, and societal well-being. The goal should be to create an AI-enhanced future that amplifies human potential rather than diminishing it, fostering a landscape that is more informed, inclusive, and ethically sound.
Author: Biswajit Biswas, Chief Data Scientist, Tata Elxsi